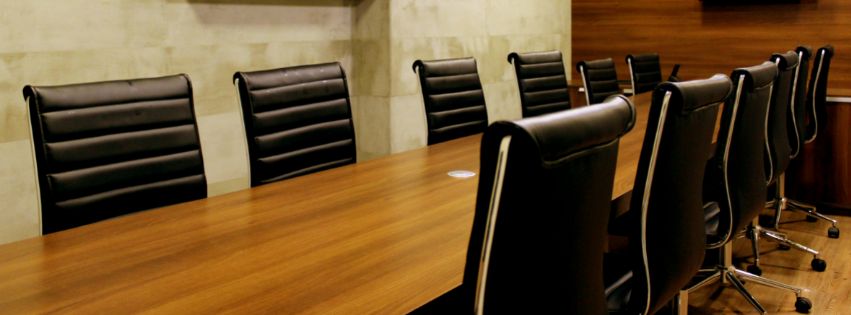
The advent of AI has raised questions about how companies can best utilize these new technologies. Between hardware requirements and privacy concerns, many boards struggle to develop a sensible AI policy. Uncertainty about Open-source AI and its unique aspects is compounding the issue. Yet, open-source AI can speed up the journey to a secure and controllable AI deployment, which is vital if you build a controlled and privacy-first AI policy. Thus, let us dive into open-source AI.
The Power of Open-Source AI
Open-source AI models offer a unique blend of opportunities and challenges that board members should understand deeply to guide strategic decisions effectively. Unlike proprietary AI systems, open-source models provide organizations with absolute transparency and control, which is increasingly vital in today’s digital landscape. This transparency means that companies can inspect the code, adjust it to their specific needs, and deploy it in environments prioritizing data privacy and security. For enterprises handling sensitive information, the ability to run AI locally without sending data to third-party cloud services is a game changer. It reduces the risk of data leakage and aligns with the growing demand for digital sovereignty and regulatory compliance. This control over data and infrastructure reflects a broader strategic imperative: maintaining ownership over critical technology assets rather than outsourcing them to external vendors.
With Power Come Costs
However, the experience of running these models locally is not without its complexities. Setting up open-source AI requires considerable technical expertise and infrastructure investment. Unlike cloud-based AI services, which spread hardware demand and maintenance over multiple customers, open-source AI demands robust computational resources. These resources often include high-performance GPUs and specialized software environments. Similarly, the model installation and configuration process can be intricate. It involves downloading large models, sometimes several gigabytes in size, and configuring them to run efficiently on local or private cloud systems. Tools like Ollama have emerged to simplify this process, offering command-line interfaces that make it easier to download and run models such as Mistral, known for its balance of performance and size. Yet, even with such tools, organizations must consider ongoing maintenance, tuning, and potential troubleshooting that come with managing AI infrastructure in-house.
Strategic Open-Source
Adopting open-source AI models should align with the organization’s broader digital transformation goals. Boards must weigh the benefits of cost savings, customization, and data privacy against the operational demands and risks. Open-source AI can be more cost-effective over time because it avoids the recurring usage fees typical of cloud-hosted AI services, which charge based on query volume and GPU time. This financial advantage particularly appeals to companies with high-volume or sensitive AI workloads. Moreover, open-source fosters innovation by enabling internal teams to experiment, customize, and improve models collaboratively, often with support from vibrant developer communities. This dynamic can accelerate product development cycles and create competitive differentiation. However, boards should also consider the risks related to cybersecurity, model governance, and regulatory compliance. As AI becomes more embedded in core business processes, ensuring robust oversight and ethical use is essential to avoid reputational and legal pitfalls.
AI Governance
The governance of AI, including open-source AI, demands a proactive and informed approach at the board level. AI’s rapid evolution and potential impact on business models require boards to engage deeply with the technology’s capabilities and limitations. According to recent insights, many boards currently lack sufficient understanding of AI’s possibilities and risks, which can hinder effective oversight. To address this, boards should prioritize AI literacy, ensuring that directors and executives receive ongoing education on AI trends, ethical considerations, and regulatory developments. This knowledge enables them to define clear AI strategies that integrate with the company’s mission and risk appetite. Establishing a technology committee that oversees AI ethics, strategy, and risk can help advance these goals without blocking the whole board.
Yet, AI governance goes beyond the technological aspect. Boards must also foster transparent communication channels with management and technical teams to continuously monitor AI implementation and performance. This governance framework helps balance the need for innovation speed with the imperative to manage risks prudently.
Staying in Control With Open-Source
In conclusion, the experience of running open-source AI models is a strategic journey that blends technological innovation with governance rigor. For boards, understanding the nuances of open-source AI, from its operational demands and cost implications to its governance and security challenges, is essential for making informed decisions that drive sustainable growth. Open-source AI empowers organizations to maintain sovereignty over their data and technology, which fosters innovation and resilience in a competitive landscape. Yet, this empowerment comes with responsibilities. Organizations must invest in the right talent, infrastructure, and governance frameworks to harness AI’s full potential safely and ethically. As AI reshapes industries, boards that proactively engage these issues will position their organizations to thrive in the evolving digital economy.